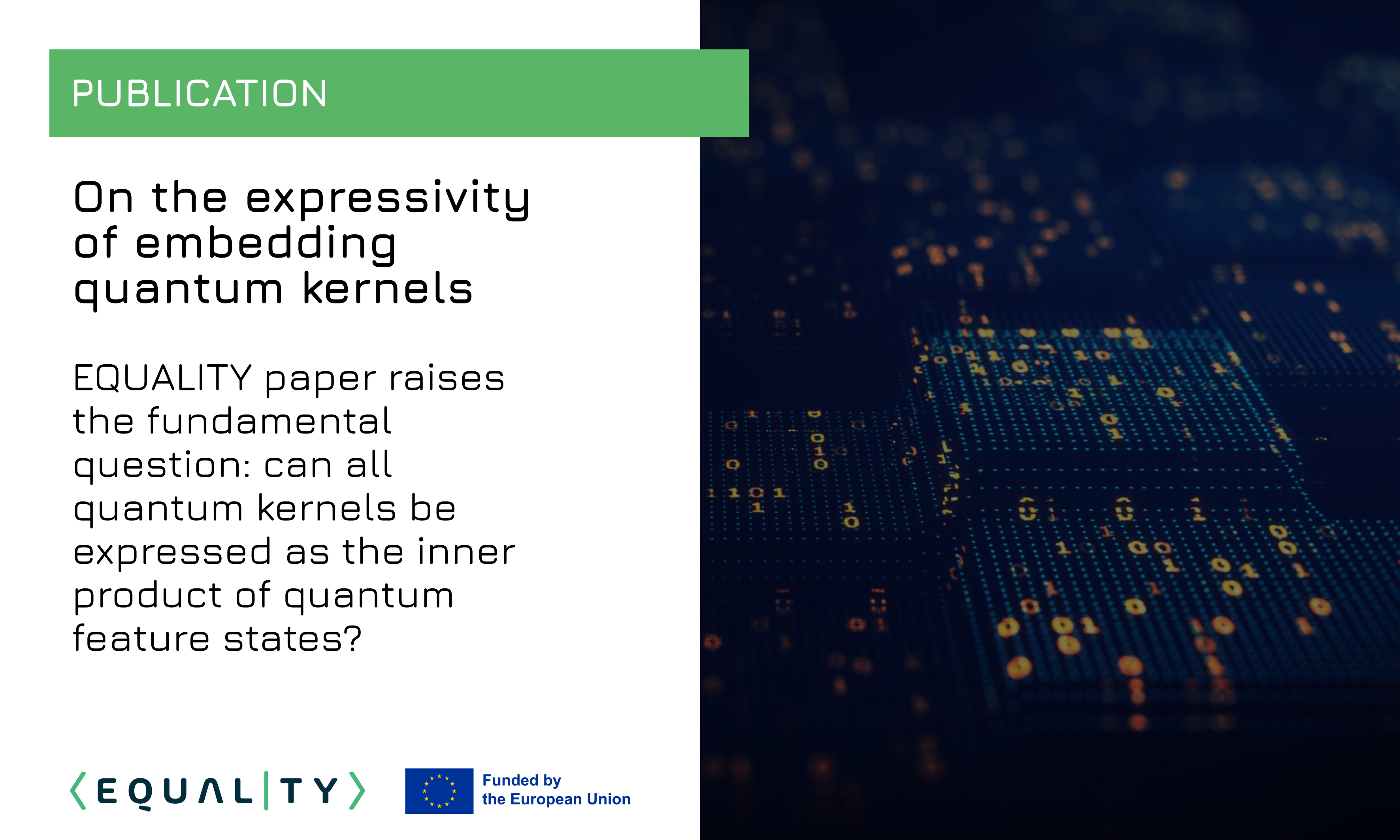
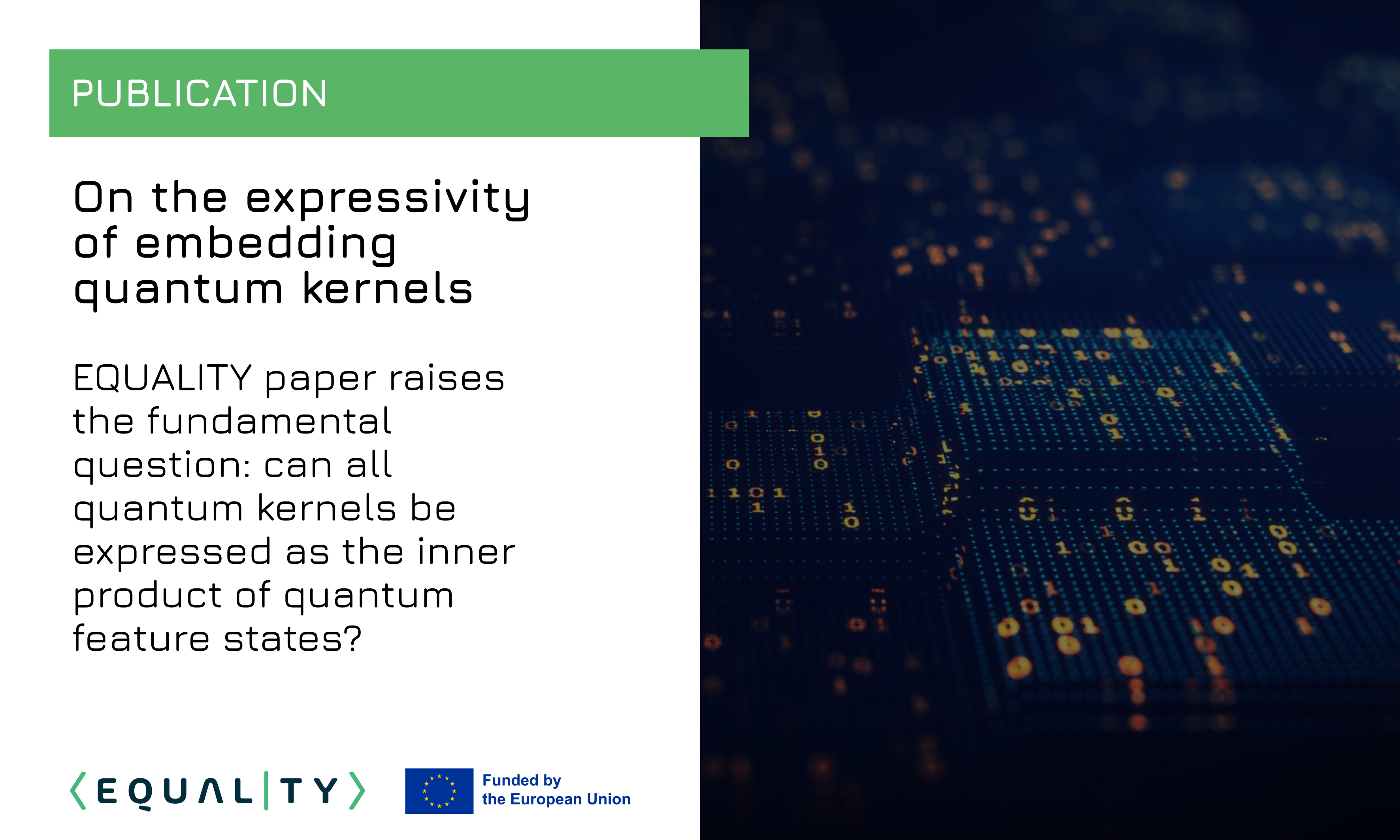
Quantum devices carry the promise of surpassing classical computers in certain computational tasks. With machine learning playing a crucial role in predictive tasks based on training data, the question arises naturally to investigate to what extent quantum computers may assist in tackling machine learning (ML) tasks.
One of the most natural connections between quantum and classical machine learning has been established in the context of kernel methods. They rely on kernels, which are inner products of feature vectors living in large feature spaces.
Their first result is positive: Invoking computational universality, they find that for any kernel function there always exists a corresponding quantum feature map and an embedding quantum kernel. The more operational reading of the question is concerned with efficient constructions, however.
In a second part, they formalize the question of universality of efficient embedding quantum kernels. They use the technique of random Fourier features to show that shift-invariant kernels are universal within the broad class of all kernels which allow a variant of efficient Fourier sampling.
The authors then extend this result to a new class of so-called composition kernels, which are shown to also contain projected quantum kernels introduced in recent works. After proving the universality of embedding quantum kernels for both shift-invariant and composition kernels, they identify the directions towards new, more exotic, and unexplored quantum kernel families, for which it remains open whether they correspond to efficient embedding quantum kernels.
Read the paper by clicking on the link below.